Table of Contents
Generative AI represents a significant evolution in artificial intelligence technology, capable of producing various types of content such as text, imagery, audio, and synthetic data. Its most recent developments, particularly in language models like ChatGPT, have garnered widespread attention due to their ability to generate human-like text, including essays, poetry, and jokes, in response to prompts.
These advances have surpassed previous achievements in AI, such as those in computer vision, where AI was known for creating aged features in images or transforming selfies into Renaissance-style drawings.
The essence of generative AI lies in its ability to learn and replicate the 'grammar' or underlying patterns of different types of data, not just in images but also in language, software code, and chemicals, among others.
This ability has opened up numerous practical applications across various industries, transforming content production and offering new tools for developers and users alike.
From creating more engaging and personalized content to automating repetitive tasks, generative AI is reshaping how we interact with and leverage technology in our daily lives and work.
Understanding Generative AI
Users can input a range of prompts to create new content, including text, photographs, videos, audio, code, 3D designs, and other media, using generative artificial intelligence, or GenAI. It is "trained" using pre-existing online materials and artifacts.
As generative AI is trained on additional data, it develops. It uses artificial intelligence (AI) models and algorithms that are trained on big, unlabeled data sets, which demand sophisticated mathematics and a lot of processing power to produce. These sets of data teach the AI to forecast results in ways that resemble actions or creations made by humans.
The proliferation of use cases for generative AI can be attributed in large part to the fact that people can now trigger AI with natural language. AI generators are currently being employed as writing, research, coding, designing, and other industry partners.
How Generative AI Models Work?
When people discussed artificial intelligence (AI) prior to the recent explosion in generative AI, they usually discussed machine-learning models, which are algorithms that can learn to make predictions based on data.
These models are trained, for example, on millions of samples to forecast if a specific X-ray indicates the presence of a tumour or whether a specific borrower is likely to miss payments on a loan.
Rather than predicting anything based on a pre-existing dataset, generative AI might be compared to a machine-learning model that has been trained to generate new data. A generative AI system is one that picks up the ability to create additional objects that resemble the training set of data.
The lines separating generative AI from other forms of AI can become hazy when it comes to the actual hardware that powers them. According to Phillip Isola, an associate professor of electrical engineering and computer science at MIT and a member of the Computer Science and Artificial Intelligence Laboratory (CSAIL), "the same algorithms can often be used for both."
Furthermore, the technology itself isn't really new, despite the excitement around the launch of ChatGPT and its equivalents. These potent machine-learning models are based on over 50 years of study and computational developments.
World’s top companies use ClanX to build AI enabled products to transform their business — expertly executed in weeks, not months.
How Do You Evaluate Generative AI Models?
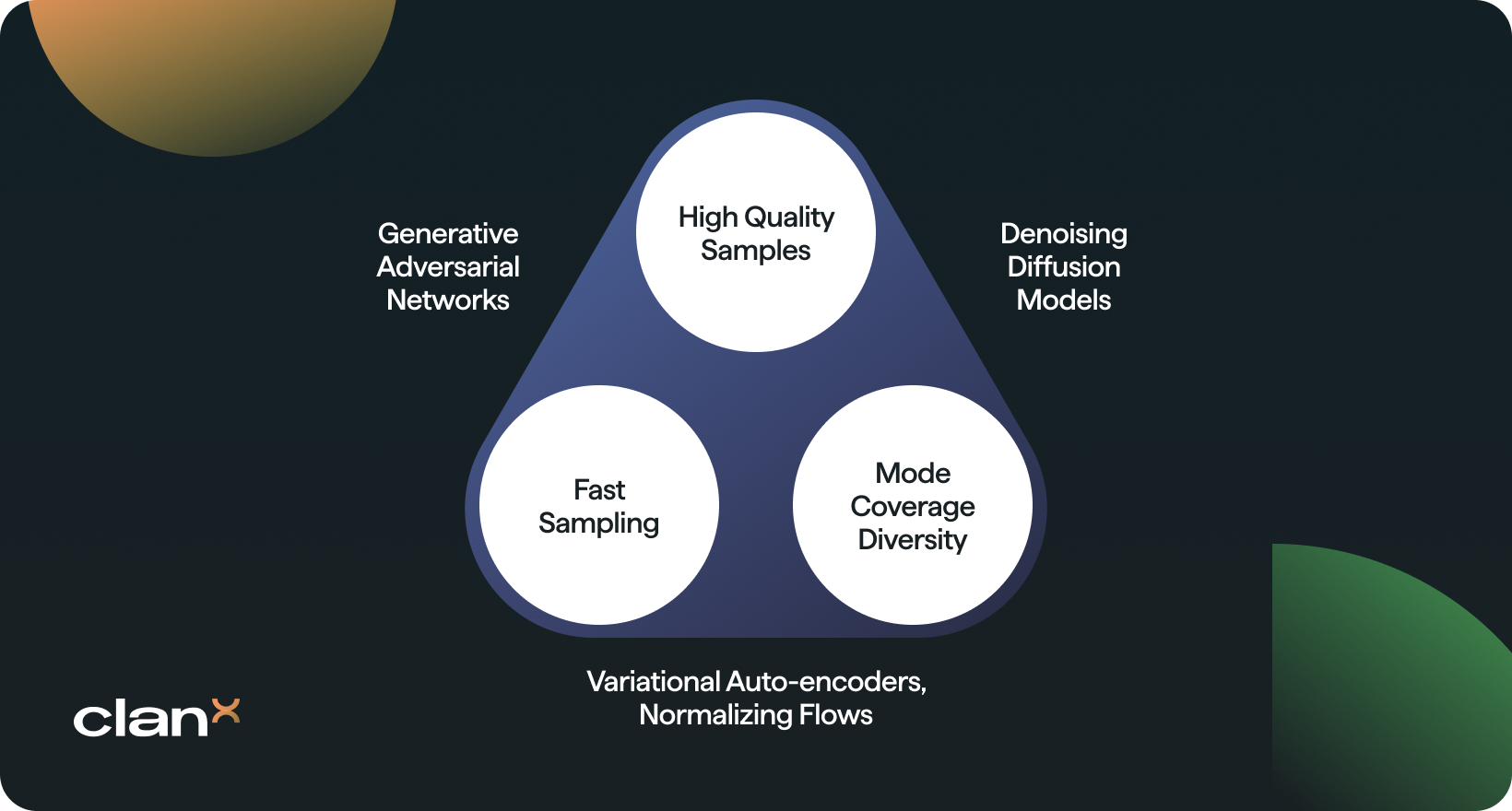
Three essential elements are needed for a generative AI model to be successful:
- Quality: Having high-quality generated outputs is essential, especially for apps that communicate directly with users. For example, when it comes to speech creation, poor quality speech is difficult to understand. In the same way, when taking pictures, the goal is to produce images that look exactly like real photos.
- Diversity: Without compromising the quality of its generation, a good generative model captures the minority modes in its data distribution. As a result, the trained models' biases are minimizes.
- Speed: For use in processes for content creation, many interactive applications—like real-time picture editing—need to be generated quickly.
Historical Development

The development of neural networks, or interconnected layers of "neurons" that process and learn from data like the human brain, was made possible by advances in deep learning and machine learning in the 2000s, which gave rise to the general artificial intelligence (GAN) movement.
Neural networks can make predictions and judgements on their own without the need for programming because they have been trained to identify patterns in datasets.
However, a particular kind of neural network known as a Generative Adversarial Network (GAN), created in 2014 by Ian Goodfellow and colleagues, is responsible for the creative potential of GenAI. By including two neural networks into the architecture—a discriminator and a generator—that compete to enhance the quality of the generated data, GANs revolutionised the creation of images.
Furthermore, additional developments like Transformers (which use natural language processing, or NPL), Variational Autoencoders (VAEs), and Recurrent Neural Networks (RNNs) started to show off AI's capacity to produce original, imaginative content.
Applications of Generative AI
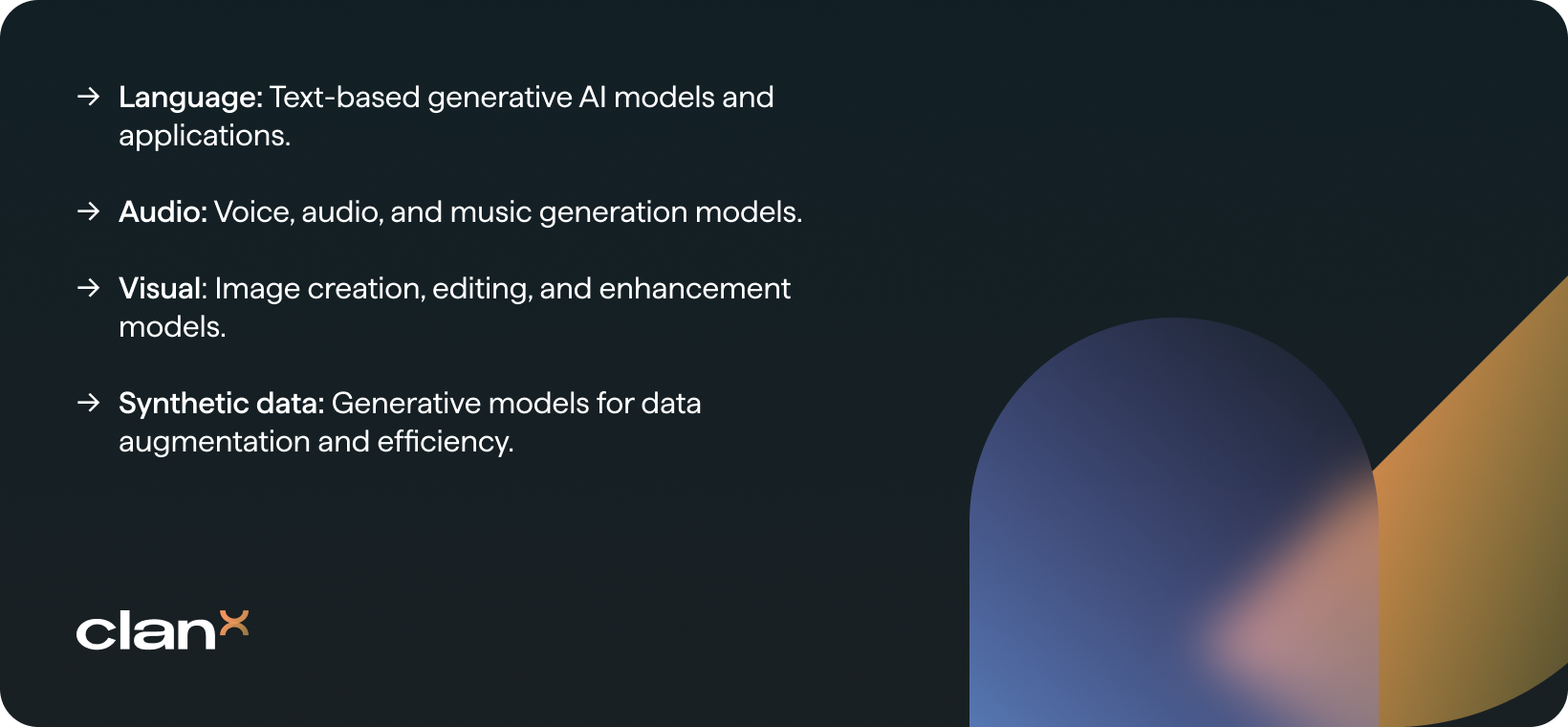
Generative AI is revolutionizing a multitude of industries with its capability to create new, original content and data. Its applications extend from enhancing creativity in art and design to advancing research in healthcare, and from transforming content generation in media to optimizing processes in business and manufacturing.
Here's a look at some of the key areas where it is making a significant impact:
- Language: Text is thought to be the most sophisticated domain and is the foundation of many generative AI models. Large language models (LLMs) are among the most well-known instances of generative models based on language. Big language models are being used for many other kinds of activities, including writing essays, writing code, translating texts, and even deciphering genetic sequences.
- Audio: Within generative AI, other developing fields include voice, audio, and music. As examples, consider models that can compose original music, identify objects in films and generate sound effects for them, and compose songs and short audio clips with text inputs.
- Visual: The field of images is one in which generative AI is most widely used. This includes making videos, graphs, avatars, and other types of illustrations in three dimensions. It's possible to create images in a variety of artistic styles and use editing and modification techniques to produce visuals.
- Synthetic data: When real data is unavailable, restricted, or just not adequate to address corner cases with the maximum level of accuracy, synthetic data comes in quite handy for training AI models. One of the most effective ways to address the data difficulties faced by many businesses is to use generative models to create synthetic data.
The impact of generative models is wide-reaching, and their applications are only growing. Here are just a few examples of how the areas of transportation, the natural sciences, and entertainment are being advanced and transformed by generative AI.
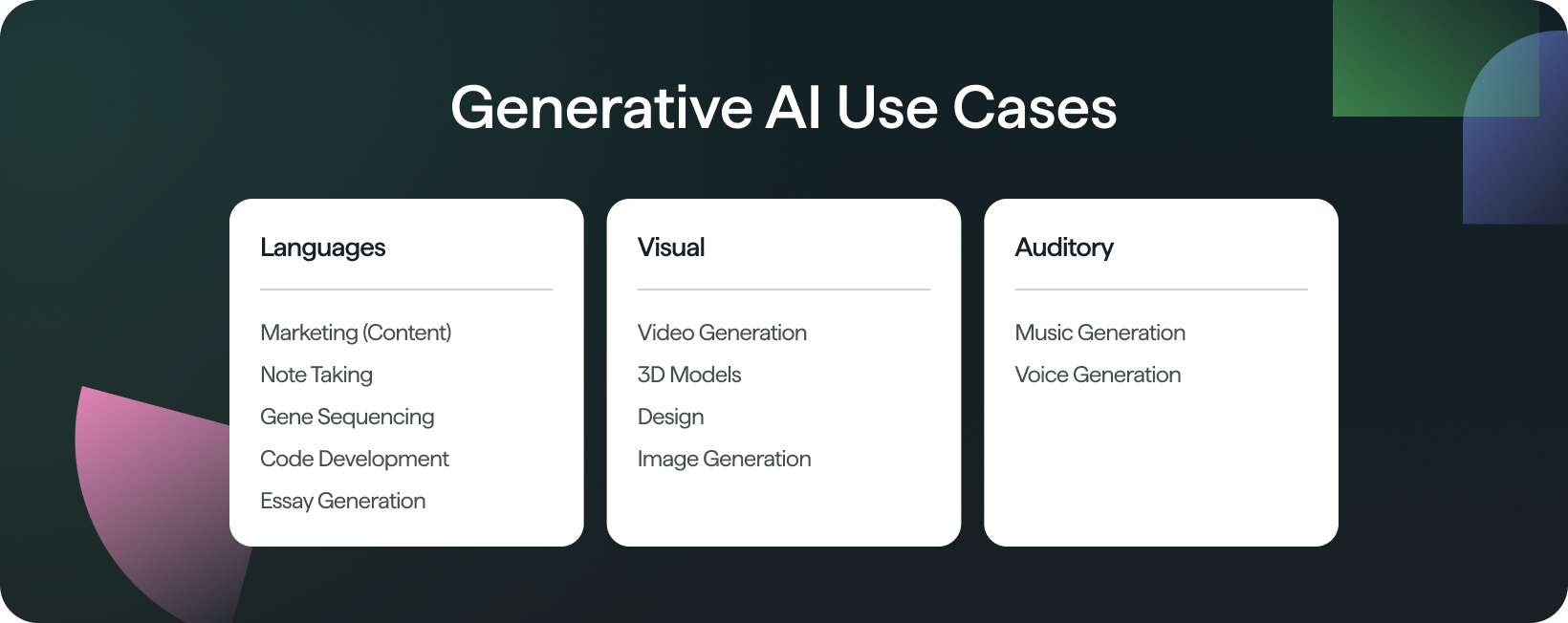
- Generative AI is anticipated to assist in the creation of 3D environments and models for simulations and automobile development in the automotive sector. Autonomous vehicles are also being trained with synthetic data.
- Road testing an autonomous vehicle's capabilities in a realistic 3D environment reduces risk and overhead while increasing safety, efficiency, and flexibility.
- Generative AI has enormous benefits for the natural sciences field. Generative models have the potential to facilitate drug development in the healthcare sector by generating novel protein sequences. The automation of processes like genomic analysis, medical imaging, medical coding, and scribing can also be advantageous to practitioners.
- Generative models, on the other hand, can be utilised in the weather industry to build planet simulations and aid in precise weather forecasting and natural catastrophe prediction. Scientists can forecast natural disasters and improve preparation with the use of these technologies, which can contribute to safer settings for the general public.
- Generative AI models can be utilised by various entertainment industries, such as video games, movies, animation, world-building, and virtual reality, to facilitate the generation of content more efficiently. Generative models are being used by creators as an additional creative tool.
Refer companies, earn a share of ClanX’s fees.
What are the Benefits of Generative AI?
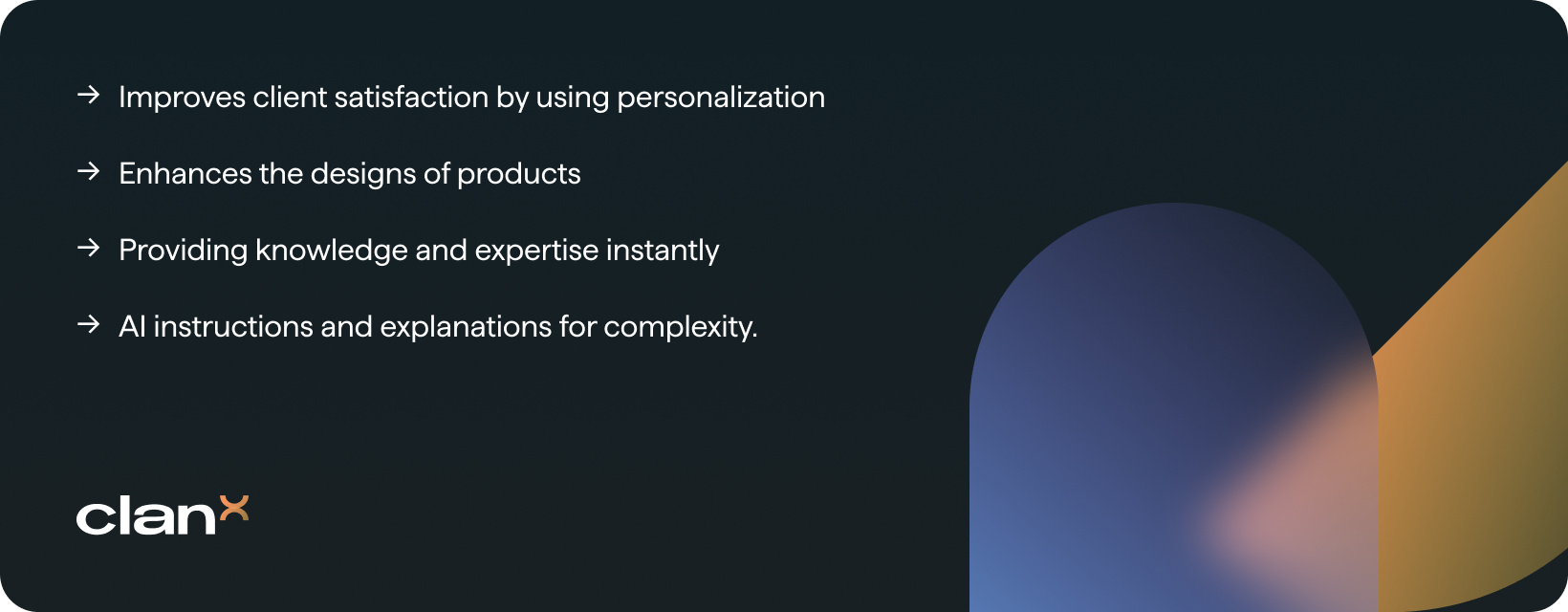
Innovates and automates the creation of content. Businesses can use generative AI to produce a variety of content, including blog entries, social media posts, marketing copy, and graphic design.
AI tools can be programmed to do certain tasks, including building a landing page that caters to the wants and solutions of your target audience. AI tools can also be used to generate new ideas and refine them based on feedback, which promotes greater innovation.
- Improves client satisfaction by using personalization: Through personalising consumer encounters, AI can enhance corporate processes. Using the consumer information you gather, AI may learn about your business and its offerings and use generative AI to produce individualised experiences.
- Enhances the designs of products: Businesses can increase efficiency and product design innovation with the use of generative AI. AI can spot market trends and customer behaviour by analysing vast amounts of data, which helps businesses lower risk and make smarter decisions.
- Providing knowledge and expertise instantly: Questions on a wide range of subjects can have expert-level responses from generative AI. Everyone now has access to a potent knowledge engine, thanks to this. AI tutors can help students learn more effectively. Experts can make judgments with the help of AI insights.
- Additionally, anyone can employ AI instructions and explanations for tasks or subjects that are complex. The understanding of generative AI is not yet perfect, but it is developing. In many domains, it might soon equal or even exceed human competence. Knowledge about AI is already widely available.
Building a Generative AI Solution
To build a successful generative AI system, there are several critical steps to follow. Let's break them down:
- Define the Problem and Objective Setting: This step involves clearly outlining the goals for your generative AI project. Ask questions like: What specific content do you aim to generate? What are the desired features or characteristics of this content? How will you measure success?
- Defining clear, measurable objectives is crucial for guiding the development process and evaluating the outcome.
- Data Collection and Management: The quality and relevance of your dataset are paramount. Collect a comprehensive dataset that accurately represents the domain you're targeting.
- Data Processing and Labeling: Prepare your data for the model. This includes cleaning (removing irrelevant or corrupt data), filtering (selecting the most relevant data), augmenting (increasing the diversity of your dataset), and transforming data into a format suitable for your model.
- Depending on your model's requirements, labeling the data with tags or categories might be necessary.
- Choosing a Foundational Model: Select the right algorithm or model type. Common choices for generative AI include transformers (like GPT), Generative Adversarial Networks (GANs), and variational autoencoders. Consider the model's architecture, hyperparameters, and optimization methods to fit your specific needs.
- Model Training and Fine-Tuning: Train your model on the prepared dataset. This step requires suitable computing resources and frameworks. Fine-tuning involves adjusting various parameters (like learning rate or regularization techniques) to optimize the model's performance and output quality.
- Model Evaluation and Refinement: Evaluate your model using relevant metrics, which may include accuracy, diversity, coherence, creativity, etc. Refine the model based on this evaluation, which could mean adding more data, altering the model structure, or experimenting with different training techniques.
- Deployment and Monitoring: Deploy your model in the intended environment, which could be a web application, mobile app, or a cloud-based service. Monitor its performance, reliability, and security continuously. Be prepared to update and maintain the model to adapt to new data, user feedback, or changing requirements.
Each step is vital to creating a robust, effective generative AI system. It's a process that requires careful planning, execution, and ongoing management to ensure the AI performs as intended and remains relevant and secure over time.
Land your dream engineering job using ChatGPT
Best Practices for Writing GenAI Prompts
An input phrase or hint that directs the output of a GenAI model is called a prompt. Prompts are used by GenAI models to produce fresh, creative content that is statistically aligned with the prompt's needs and context.
The same fundamental ideas underpin the best practices for creating text, image, audio, and video prompts, even though the specifics of each form of prompt reflect the kind of output that is intended.
- Be Precise: The response will probably be more customized if the prompt is more precise and comprehensive.
- Provide Context: Context clears up confusion and aids in the model's production of outputs that reflect the intent of the prompter.
- Avoid Leading Questions: It's crucial to provide prompts that are impartial and free of prejudiced data.
- Reframe & Iterate Prompts: Try rephrasing the query or altering the base multimedia sample if the model doesn't provide a helpful response the first time.
- Adjust Temperature Configurations: There are AI platforms that let users change the temperature. More predictable results are produced at lower temperatures, whereas more random results are produced at higher temperatures.
- Limit Response Length: Create prompts that outline restrictions, such as word or character counts for text outputs or duration limits for audio outputs when you're looking for succinct responses.
- Experiment with Multiple Prompts: More relevant results are frequently obtained by segmenting a question or instruction into multiple smaller prompts or by experimenting with alternative base pictures, audio clips, and video samples.
- Review & Revise Outputs: Since most genAI replies require editing before they can be deployed, generative AI outputs should constantly be examined. Be ready to dedicate some time to this crucial.
Ethical Considerations and Challenges
We need to be aware of the ethical and legal issues surrounding generative AI as it develops in order to prevent them from growing along with the technology.
The issue of AI control draws attention to the ever more difficult task of making sure AI upholds human values and functions to our advantage rather than against them. Concerning us now are the human biases concealed in the algorithms that drive these prevalent technologies. As technology develops, what will happen to the already dark waters of technology compliance?
The black box problem, which explains why it can be challenging to comprehend how some generative models arrive at their outputs, is another issue to take into account. How can we tell if the material we are given is impartial and free of prejudice?
This raises considerations about ethical AI usage and responsibility, as well as legal concerns around the possibility that GenAI models would produce harmful or false information.
The application of this technology in the political sphere raises grave problems, as viewers may be tricked by deepfake images of political people expressing opinions that differ from their own. This has significant ramifications for how voters' political opinions may be shaped by false information.
Furthermore, copyright and intellectual property concerns are raised by GenAI. The legal ramifications of GenAI content created using previously published works by original creatives are still unknown. specifically with regard to ownership, unlawful content in training data, and copyright infringement.
Legislators are still developing clear guidelines on how to apply current intellectual property rules to this emerging trend because these issues are still in their infancy.
Which Industries are Most Impacted By Generative AI?
By enhancing essential procedures using AI models, generative AI will have an impact on the pharmaceutical, manufacturing, media, architectural, interior design, engineering, automotive, aerospace, defence, medical, electronics, and energy sectors.
By enhancing the supporting processes that cut across numerous organisations, it will have an effect on training, software engineering, corporate communications, design, marketing, and training.
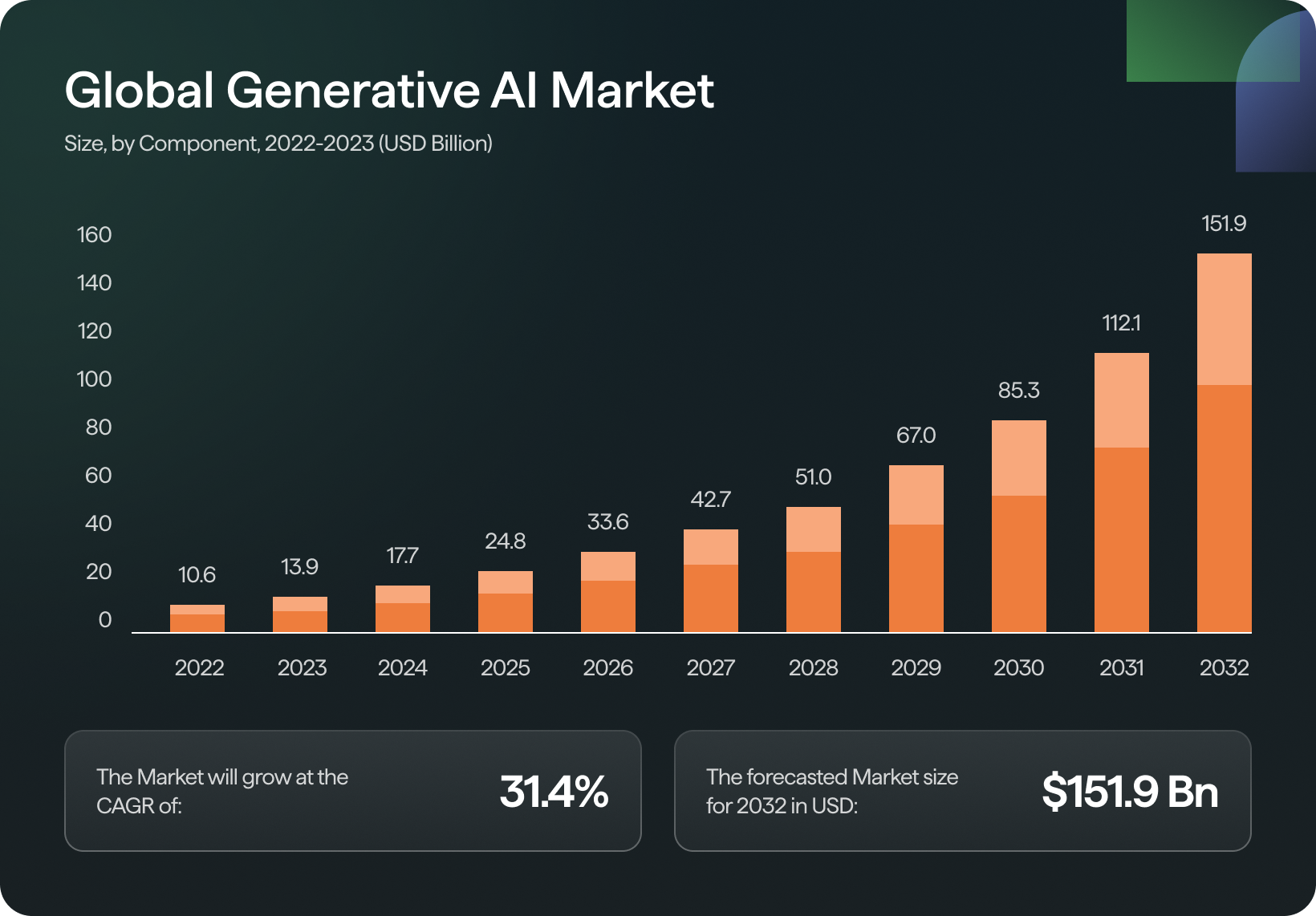
Researchers predict that by 2025, generative AI approaches will be used to systematically discover about 30% more new medications and materials, as opposed to the current zero percent. With the potential to cut costs and time associated with drug discovery, generative AI appears to be a promising development for the pharmaceutical industry.
It is projected that by 2025, thirty percent (30 %) of large organisations' outbound marketing communications will be generated synthetically, a rise from less than two percent in 2022. Personalisation and marketing material can already be produced with text generators such as GPT-3.
Generative design is used in the industrial, automotive, aerospace, and defence sectors to produce designs that are optimised to fulfil particular requirements and limitations, like those related to performance, materials, and manufacturing techniques. This generates a multitude of viable solutions that engineers can investigate, hastening the design process.
Become an AI Accelerated Engineer Using ChatGPT
Future Opportunities and Directions for Generative AI

The landscape of generative AI has traditionally been dominated by the scalability of models, where larger datasets and more complex algorithms yield better results. Predictive scaling has allowed researchers to anticipate the performance of these massive models before committing significant computational resources.
However, recent trends show an increasing focus on the emergent capabilities of AI at certain scales, with larger models not only being defined by their architecture but also by their ability to perform complex tasks, like logical reasoning.
The generative AI market, valued at $10.6 billion in 2022, is expected to grow significantly, driven by demands in creative industries for more immersive and personalized experiences. Yet, the tide is turning with smaller, more specialized models proving to be more effective in domain-specific applications.
For example, Stanford's PubMedGPT 2.75B demonstrated superior performance in medical queries over its larger counterparts, suggesting that smaller models might be preferable for niche applications, offering cost-effectiveness and reduced environmental impact.
The future of generative AI may not necessarily hinge on larger models. The trend of model distillation, as seen in Stanford's project to condense GPT-3.5's capabilities into the smaller Alpaca chatbot, suggests a shift towards more compact, efficient AI systems. This approach, along with the development of models like Dolly 2 that directly gather data from human interactions, points towards an era where smaller models could suffice for a wide array of applications, balancing innovation with ethical considerations like content authenticity and privacy.
Conclusion
With the use of different cues, generative AI is a potent technology that can produce fresh material. It can create code, tell jokes, write poetry, and do a lot more. Additionally, it is capable of learning the grammar of various data types, including chemicals, sounds, movies, and photos.
Numerous fields, including research, design, education, and content creation, can benefit from the use of generative AI. It can facilitate quicker, more intelligent, and more creative work from both users and developers. But generative AI is not without its risks and problems. These include issues with quality and reliability, legal, ethical, and societal issues, as well as collaboration and trust between humans and AI.
As a result, we must comprehend how generative AI functions, as well as what it can and cannot accomplish and how to apply it sensibly. Instead of taking the place of human intelligence and creativity, generative AI is a tool that may enhance and support them.
We can anticipate more chances and options for bettering our lives and finding solutions to our challenges as generative AI develops.
Other FAQs
What is meant by generative AI? | Artificial intelligence technology, known as "generative AI" is capable of producing text, images, audio, and synthetic data, among other kinds of content. More specifically, massive amounts of pre-existing content are fed into generative AI models in order to teach the models to generate new content. Based on a probability distribution, they learn to recognise underlying patterns in the data set and, in response to cues, generate similar patterns (or outputs based on these patterns).
Is GPT a generative AI? | Yes, Generative AI is demonstrated by OpenAI's ChatGPT. With the help of a massive language model (LLM) that was trained on a tonne of data, this AI chatbot can write prose that is similar to that of a human.
What is the difference between open AI and generative AI? | Open AI frameworks offer cooperation, flexibility, and customisation while generative AI enables marketers to produce unique, captivating content at scale. The particular marketing objectives, available resources, and personal preferences of each organisation will ultimately determine which of these two strategies is best.
Which is Google generative AI? | Make use of Google's most powerful multimodal model, Gemini. Google DeepMind's multimodal model, Gemini, can comprehend practically any input, combine many kinds of data, and produce almost any kind of output. In Vertex AI, prompt and test Gemini with text, photos, videos, or code.
Is Alexa a generative AI? | Conversational AI systems are intelligent computers that individuals may communicate with through simple dialogue. Voice-activated gadgets like the Amazon Echo are making possible the kind of fantastical encounters we've been dreaming of for decades thanks to conversational AI.
What is the main goal of generative AI? | The calculus of knowledge work automation is altered by generative AI tools, which may work with human collaborators to develop material that reflects practical work. These tools can produce writing, graphics, audio, and video that resembles human work in response to simple English text cues.
Is chatbot a generative AI? | A chatbot is a software application that can interact with humans using natural language. A chatbot can be a generative AI if it uses a generative model to produce its responses, such as ChatGPT. However, not all chatbots are generative AI, as some may use rule-based or retrieval-based methods.